Treasury Operations of Banks
First the Wikipedia definition: Treasury management (or treasury operations) includes management of an enterprise’s holdings, with the ultimate goal of managing the firm’s liquidity and mitigating its operational, financial and reputational risk. Treasury Management includes a firm’s collections, disbursements, concentration, investment and funding activities. In larger firms, it may also include trading in bonds, currencies, financial derivatives and the associated financial risk management.
For a bank, this means asset/liability management, hedging interest rate risk, managing reserve and capital requirements, etc. It is also something banks provide as a value added service for their clients.
Revenue from Treasury Operations
Banks break out segment revenues that include revenue derived from treasury operations. However, they don’t carve out how much of it was proprietary trading. This is the average quarterly revenue from treasury operations since June 2014 (in Rs. Cr.) of major Indian banks:
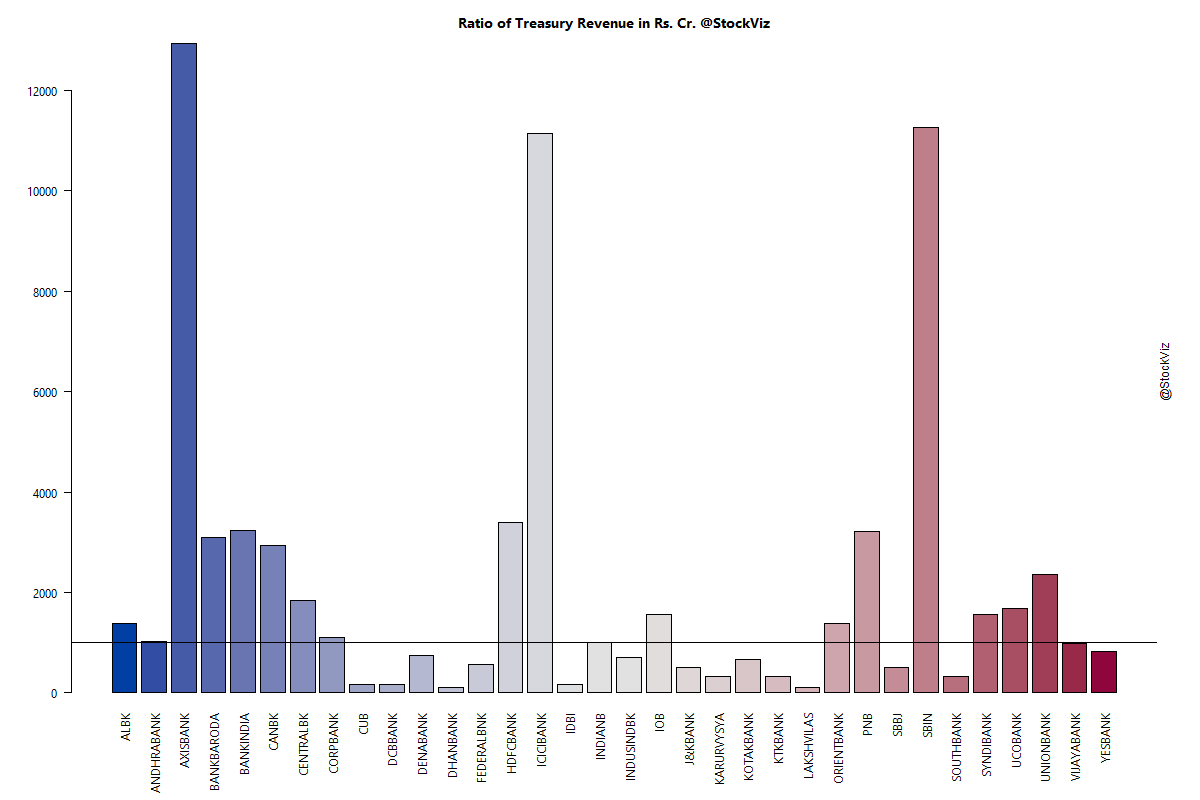
In fact, for a few of them, revenue from treasury operations exceed from those from retail banking.
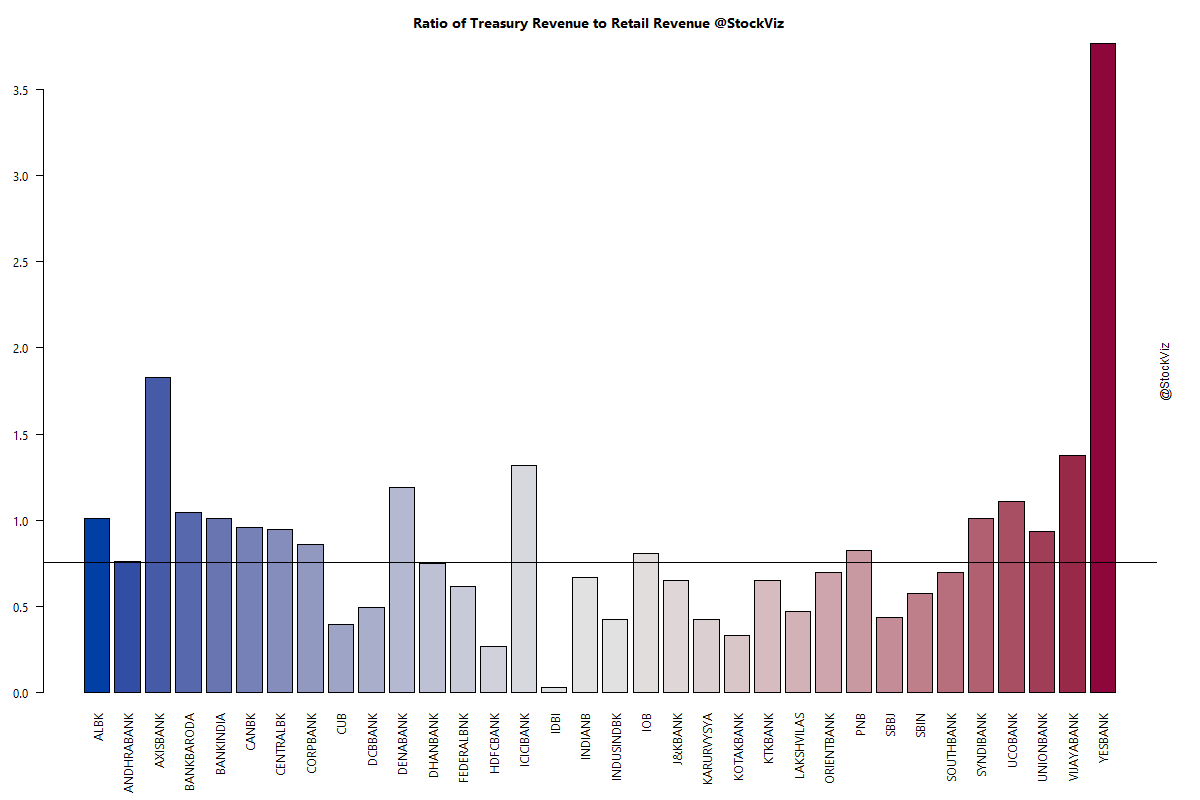
The need for more disclosure
The revenue from treasury ops is a black box. If most of that revenue is derived from prop trading, then investors need better disclosure of the risks that were taken. Recently, YESBANK got pummeled on a UBS report that raised doubts over their exposure to stressed companies. As a retail investor, we really don’t have a clue about the risks involved in holding equity in what could turn out to be a hedge fund in bank’s clothing.