Previously, we discussed how momentum itself trends and how that can be used to manage risk. Using simple moving averages showed promise when it came to some versions of our slow momentum models (see Trending Momentum Models). However, given the faster turnover of our Momos, it wasn’t a suitable approach (see Trending Momo Models).
What if we shorted the NIFTY to hedge against market-risk instead?
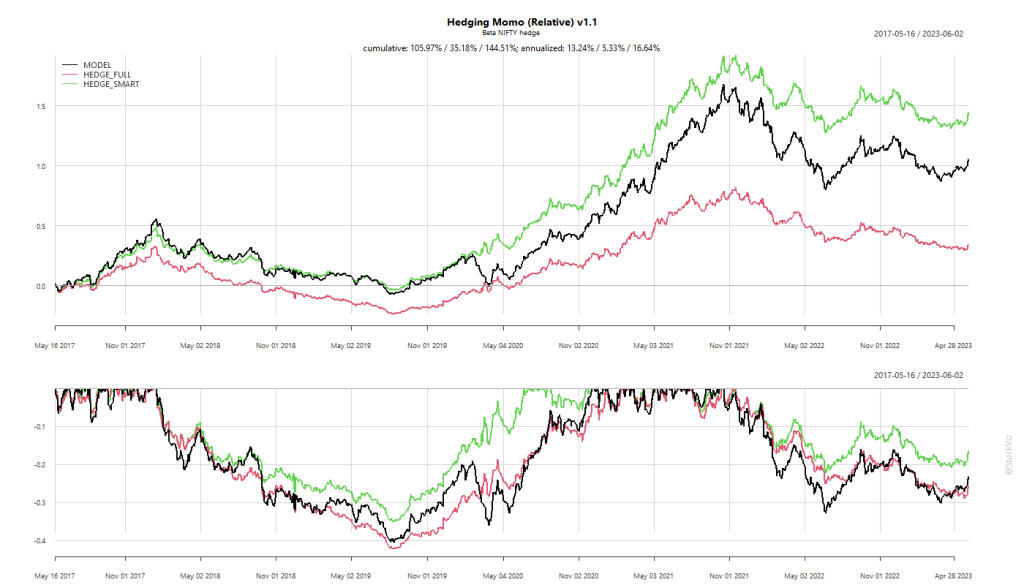
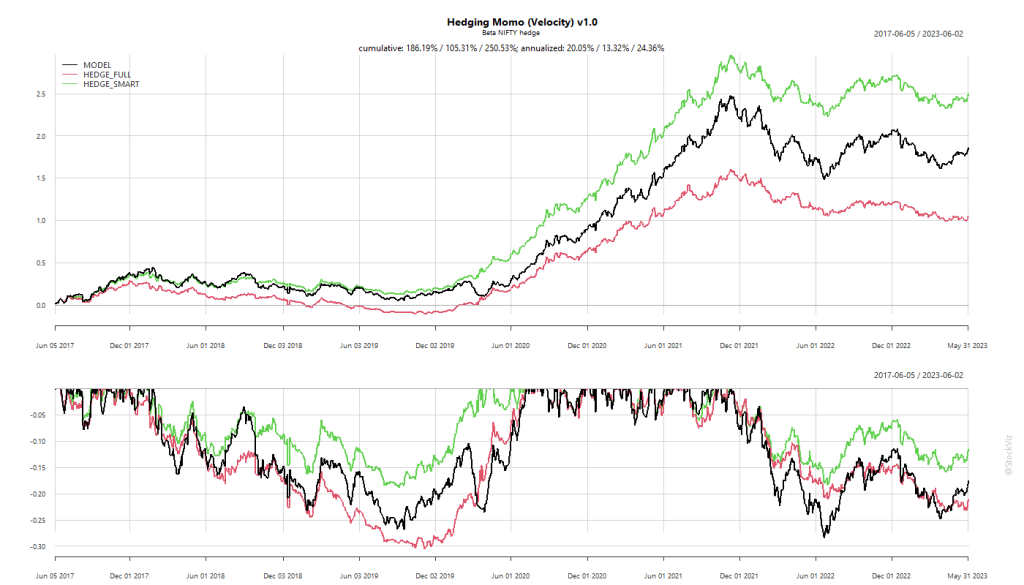
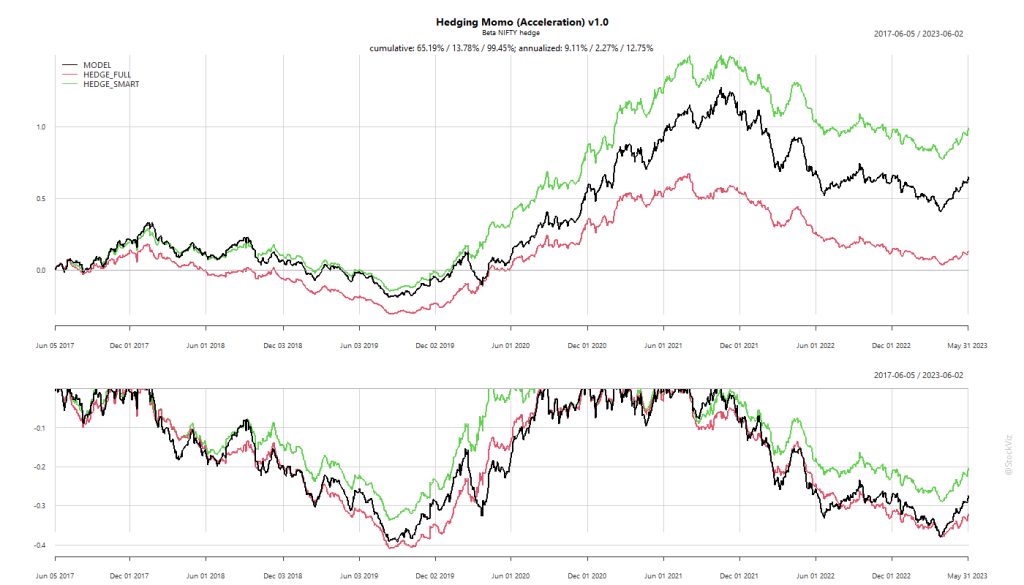
The naïve approach, tagged “HEDGE_FULL” above, shorts the NIFTY in proportion to the rolling beta of the strategy. Turns out, this is a very sub-optimal way to go about it. Hence “HEDGE_SMART”, that tries to minimize the basis risk inherent in this approach, adds about 3-4% to the strategy’s returns (likely eaten away by transaction costs & taxes) and reduces the max-drawdown by a significant amount.
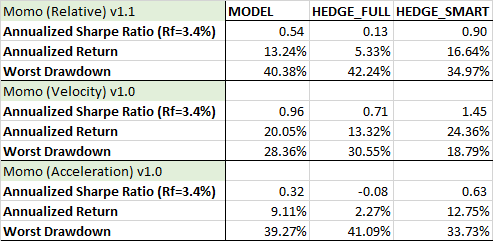
The question is whether the benefit of lower drawdowns is worth the added cost and complexity? In the case of Velocity, it could be.
Related: