Sometimes, you many not be able to directly buy an index or access a strategy because of regulatory hurdles, mandates etc… In these situations, replicating it using a basket of accessible securities might make sense.
For example, you can replicate the S&P 500 index using Indian indices: NIFTY 50, MIDCAP SELECT and NIFTY BANK.

In fact, you can do for any reference timeseries (strategies/funds) and apply leverage as desired.
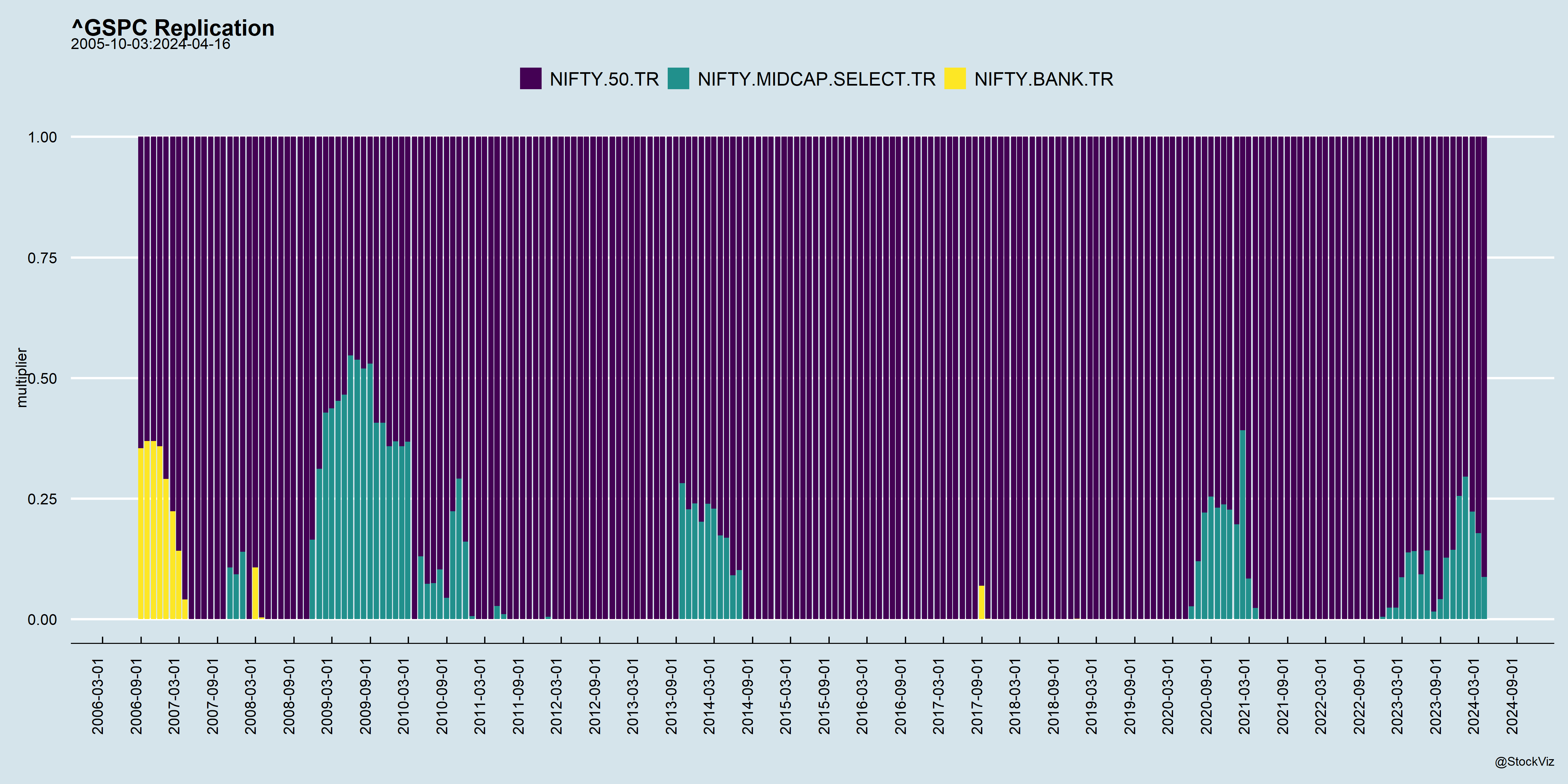
Also, the loadings give you and idea of the shifting relationship between the reference asset and the basket over time.
Code and images are on github.