In equities, buying stocks that hit their All Time Highs is a decent strategy. When combined with a trailing stop loss, it beats the NIFTY 50 index with a Sharpe of around 1.8.
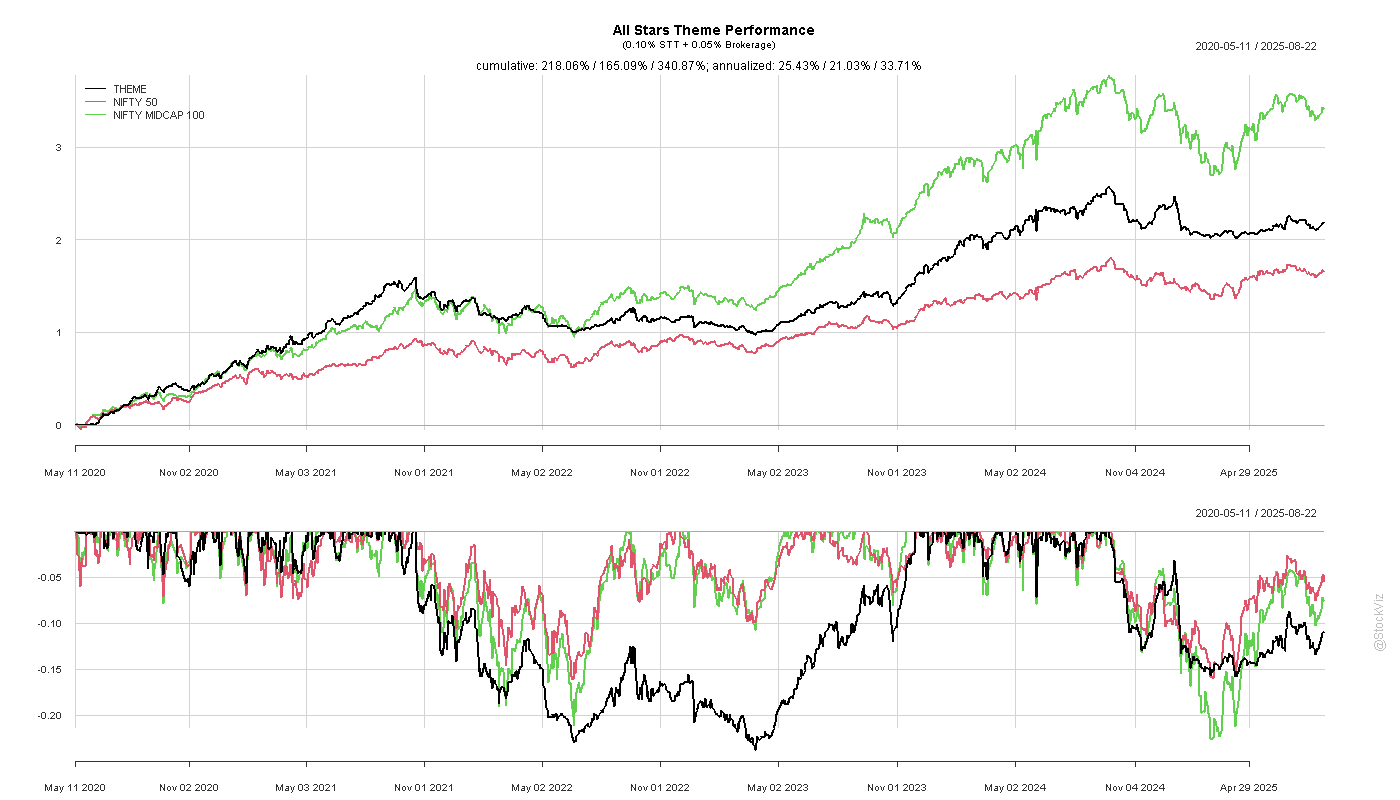
Can a similar long/short strategy work in crypto?
Since everything happens faster in crypto, we need to relax the “All-Time” constraint and consider shorter time-frames. For example, here’s the 200-day Highs stats, for returns of subsequent 1/5/10 & 20 days of L1 and L2 coins:

And here’s the same for 20-day Highs stats:

A similar thing plays out with 200- and 20-day lows.


Theoretically, you can go long coins making 20/50-day highs and go short coins making 20/50-day lows. Apply a reasonable trailing stop loss and you might have a decent strategy.
Code and charts on github.